Best Paper Award
Effects of Image Processing Operations on Adversarial Noise and Their Use in Detecting and Correcting Adversarial Images[IEICE TRANS. INF. & SYST., VOL.E105–D, NO.1 JANUARY 2022]

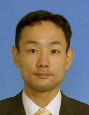

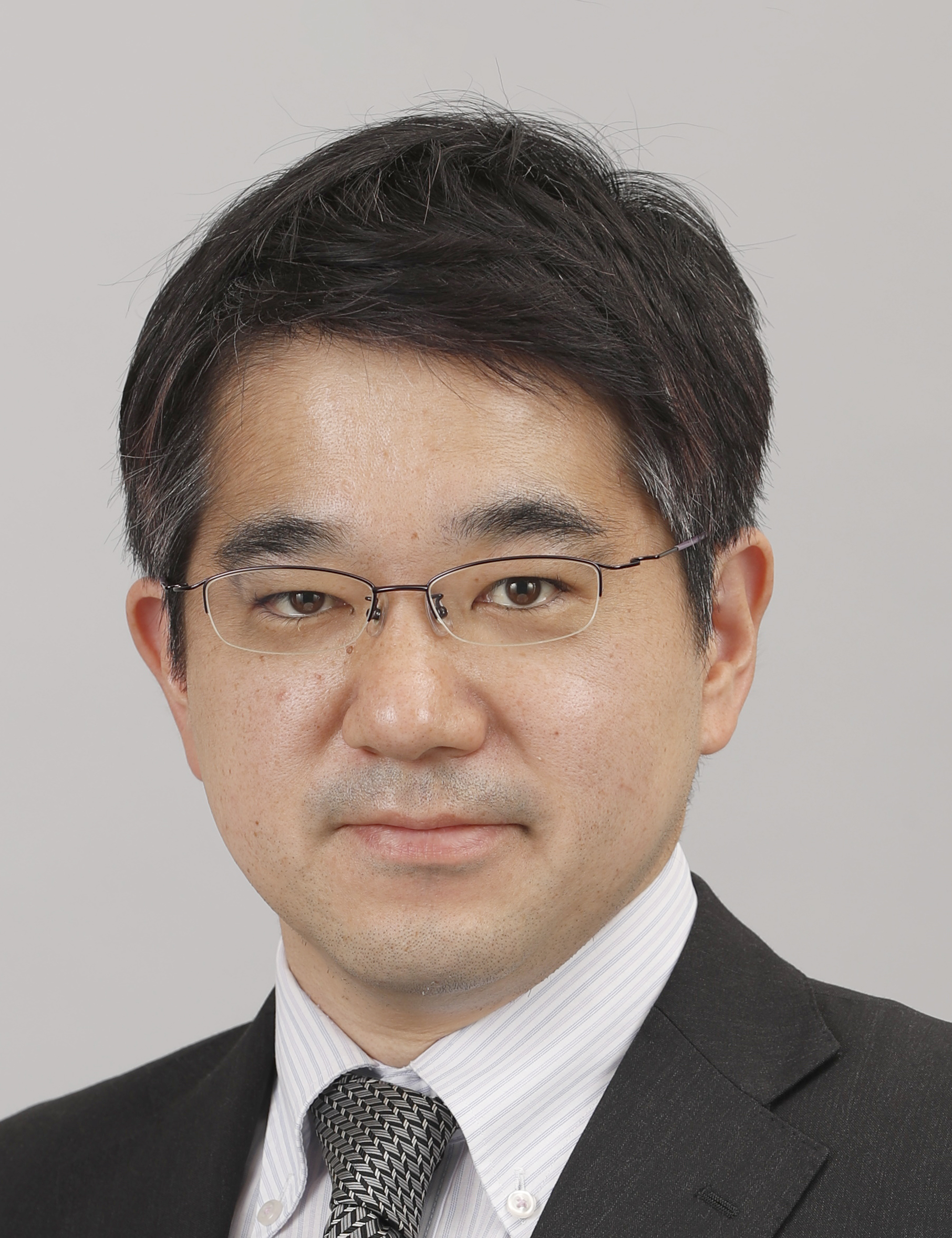
Deep neural networks (DNNs) have shown high performance in image recognition tasks and are currently being applied in various practical applications, such as autonomous driving. However, it has been pointed out that DNNs are vulnerable to adversarial attacks that add minor changes, such as noise, to input images. In addition, the existence of data poisoning attacks that add such adversarial variations to training data is also known, and research is being conducted to find and correct contaminated data to maintain the performance of DNNs.
In this paper, the authors propose (1) a standard method for constructing datasets that include adversarial data, (2) an applied method of image processing operations for detecting adversarial images, and (3) a two-stage correction process for adversarial image correction. The proposed method focuses on the effects of traditional image processing operations such as JPEG compression, Gaussian blur smoothing, rotation, and scaling on normal and adversarial images to detect adversarial image samples. For the correction of detected adversarial image data, the proposed method employs a two-stage correction process that involves label correction in the first stage and image correction in the second stage.
An evaluation experiment using a dataset containing adversarial attack data showed that the proposed method has high effectiveness in detecting and correcting adversarial images, achieving a 90% correction rate while limiting the impact on normal images to 2%.
This study addresses the critical issue of detecting and correcting adversarial samples in image datasets. The proposed detection and correction methods are innovative and demonstrate a high level of completeness as a research paper. This research has the potential to expand to modalities other than images where the existence of adversarial data is a challenge, and its content is highly deserving of a paper award.